Given a string
Output: true
Explanation: "codecs" can be made using the words from dictionary.
Output: true
Explanation: "applepenapple" can be made using "apple" and "pen" words from dictions and we have to use "pen" word two times and we are allowed to use as per problem statement.
Output: false
Explanation:"catsandog" cannot be made using the words from dictionary.
Contents
Solution 1 - using brute force approach Solution 2 - using DP memoization Solution 3 - using DP Bottom-Up approach using tabulation and memory optimized
In this approach, we will start with every word from
Say for example if the input string given is "cscode" and word dictionary is {"code", "cs"}
-
We start with 1st word
"code" from dictionary, and"cscode" is not starting with this word, so we stop exploring this path. -
Then we start with 2nd word
"cs" from dictionary, and"cscode" starts with this word, so we continue to explore this path. Since the input string starts with word "cs", we take the remainder string "code" and continue this process.-
Again we start with 1st word
"code" from dictionary, and remainder substring"code" starts with this letter, so we can return true from step.
-
Again we start with 1st word
If we look at the decision tree for all possible combinations, it will look like below diagram.
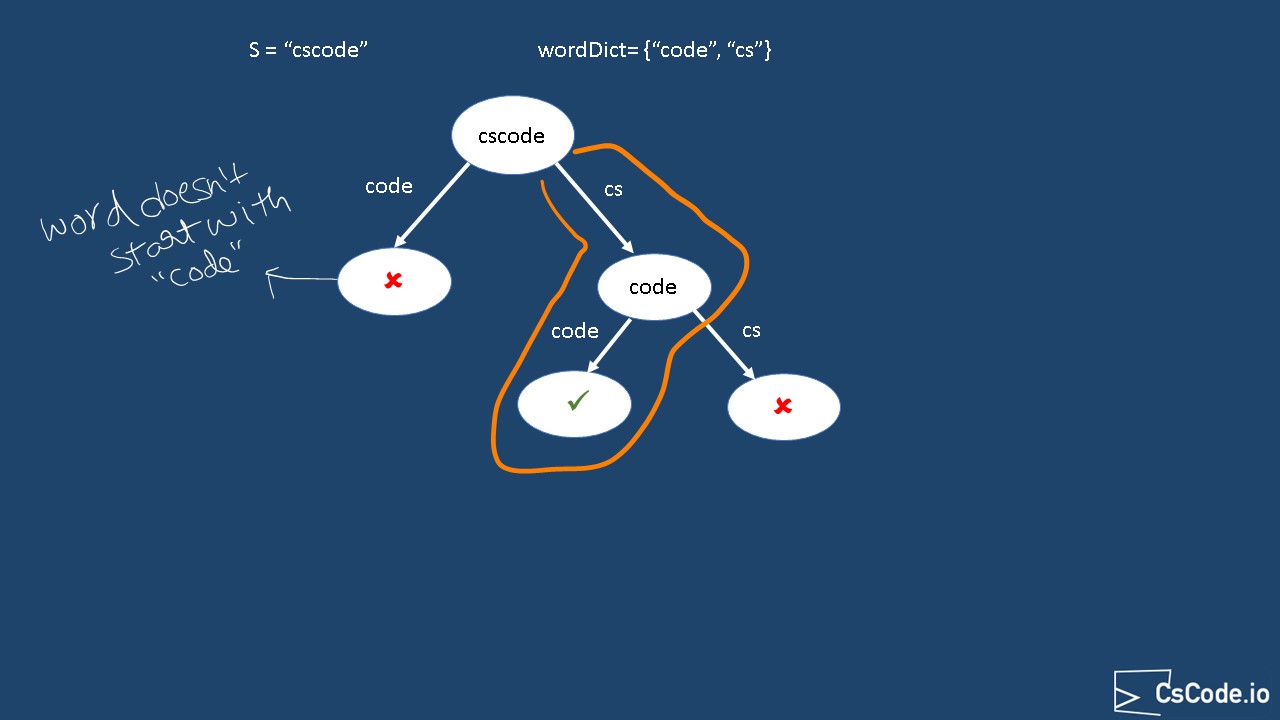
-
We will create a function
bruteForceApproach that takes input target strings and array of stringswordDict . -
Base cases are:
-
If the input string's length is 0, return
true , because when we are recursively calling the function, substrings length will become 0 only if we can make up the target string using the words fromwordDict .
-
If the input string's length is 0, return
-
For every word in
wordDict , check if the the target strings starts with that word or not.-
If the target string starts with the word, then take the remainder substring and call
bruteForceApproach recursively. -
If the target string doesn't start with the word, return
false .
-
If the target string starts with the word, then take the remainder substring and call
Complexity Analysis:
Time complexity: Above code runs in O(nm * m) time where
The height of the decision will be
Space complexity: O(m2) since we calling the function recursively, this is memory used for
remainder substring and number of recursive calls in the stack.
From the above brute force approach, we can notice that there are some overlapping problems.
For example, consider target
-
If we construct a decision tree to see the possibilities whether the string can be made using the dictionary of words
, it looks like below diagram and as you can see highlighted sub problems are overlapping.
-
So, in this approach, we will be caching sub problem result into a
HashMap with input string as key and its result as value. When a sub problem is repeated and found in cache, we will return it, otherwise we will call the function recursively and cache its result.
Complexity Analysis:
Time complexity: Above code runs in O(n * m2) time where
Space complexity: O(m2) for the cache we are maintaining (m) , stack for recursive calls and the substring operation (m * m).
In this approach we will look at solving the problem using dynamic programming using tabulation caching mechanism.
In this solution, we are going to create a temporary array with size as
-
To start with, at the right end of cache, we will store
true , this is the base case, if we reach end of array that means target strings can be made up using the words fromwordDict . -
Then, for each letter from right to left, check if that substring exists in the
wordDict if it exists, then copy cache value from end index of the substring.cache[i] = cache [i + length(word)]; The reason we do that is because, consider this examples = "abcd" andwordDict = {"ab", "cd"} - We start with cache as [false, false, false, false, true]
- When i= 3, neither of words "ab" or "cd" can fit from this index.
-
When i= 2, word "cd" can fit from this index. So, we update the cache:
cache[3] = cache[3 + length("cd")]; = cache[3+2]; = cache[5]; Now, the cache becomes [false, false, true, false, true]. This means that there is a bridge from current substring and from the ending index of current substring. - When i= 1, neither of words "ab" or "cd" can fit from this index.
-
When i= 0, word "ab" can fit from this index. So, we update the cache:
cache[0] = cache[0 + length("ab")]; = cache[2]; Now, the cache becomes [true, false, true, false, true]. This means that there is a bridge from current substring "ab" and from the ending index of current substring "ab" which is "cd". -
Finally we will return the value at 0th index. So, our answer will be
true .
Complexity Analysis:
Time complexity: Above code runs in O(n * m2) time where
Space complexity: O(m) for the cache we are maintaining that is of input string length.
Above implementations source code can be found at