Contents
How does Dynamic Programming work? Types of applying dynamic programming Solving Fibonacci using brute-force approach (Inefficient solution) Solving Fibonacci using Memoization (Optimized solution) Solving Fibonacci using Tabulation (Optimized solution) Solving Fibonacci using Tabulation and memory optimized (Efficient solution)
Dynamic programming works by splitting main problem into sub problems. Then, find optimal solutions to the sub problems. When the solution to sub problem is found, cache that result, and re-use it when a overlapping sub problem is occurred.
When a problem's solution depends on it's sub problem's solution, you can solve such problems using recursion, but often the draw back with recursion is that it occupies more memory to store function variables and constants into stack, as these values are kept in stack until the function returned output back to its parent function call. There is a limitation on the stack memory in the system, so it makes less efficient if the call stack is large.
We can apply dynamic programming technique if the sub problems are overlapping, there are other problems such as binary search will fall into similar pattern, that you reduce input scope in each iteration, but in each iteration input is unique and there is no overlapping of input.
For example, say we want to compute
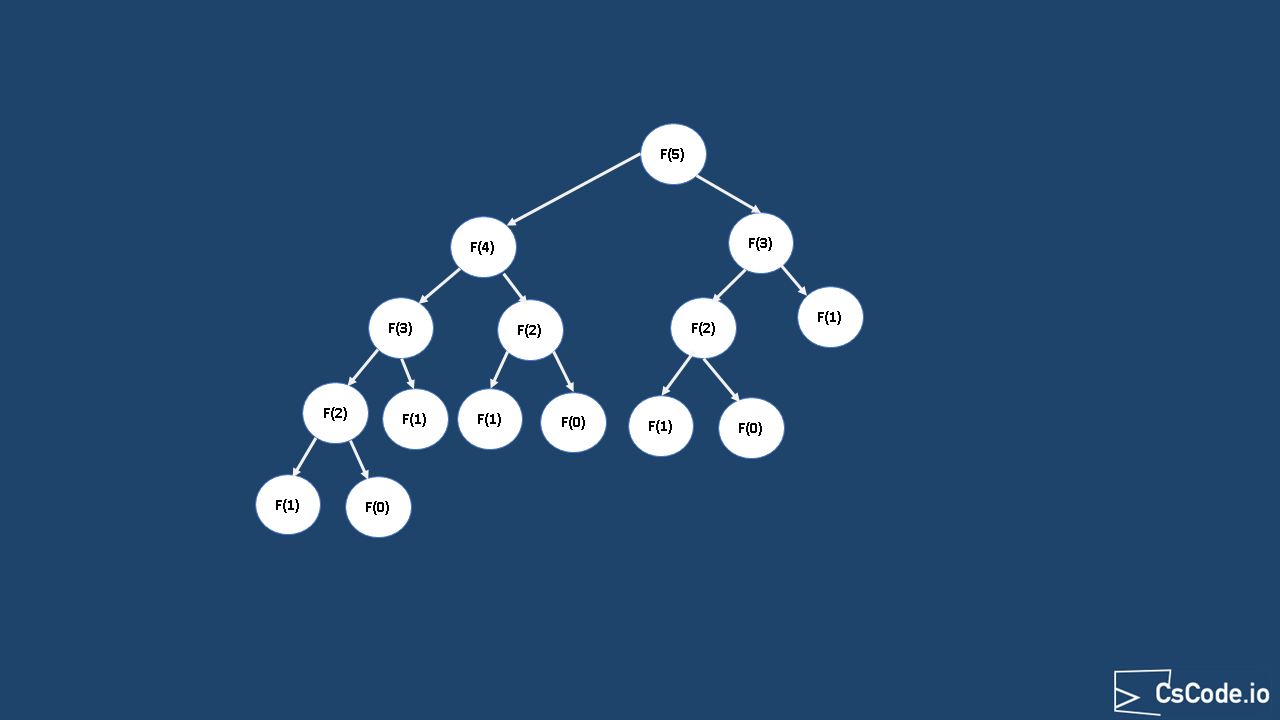
If we have to compute fibonacci sequence without dynamic programming, time complexity will be exponential O (2n)
as there are two sub problems at every step
If you notice above diagram, there are some overlapping problems, for example
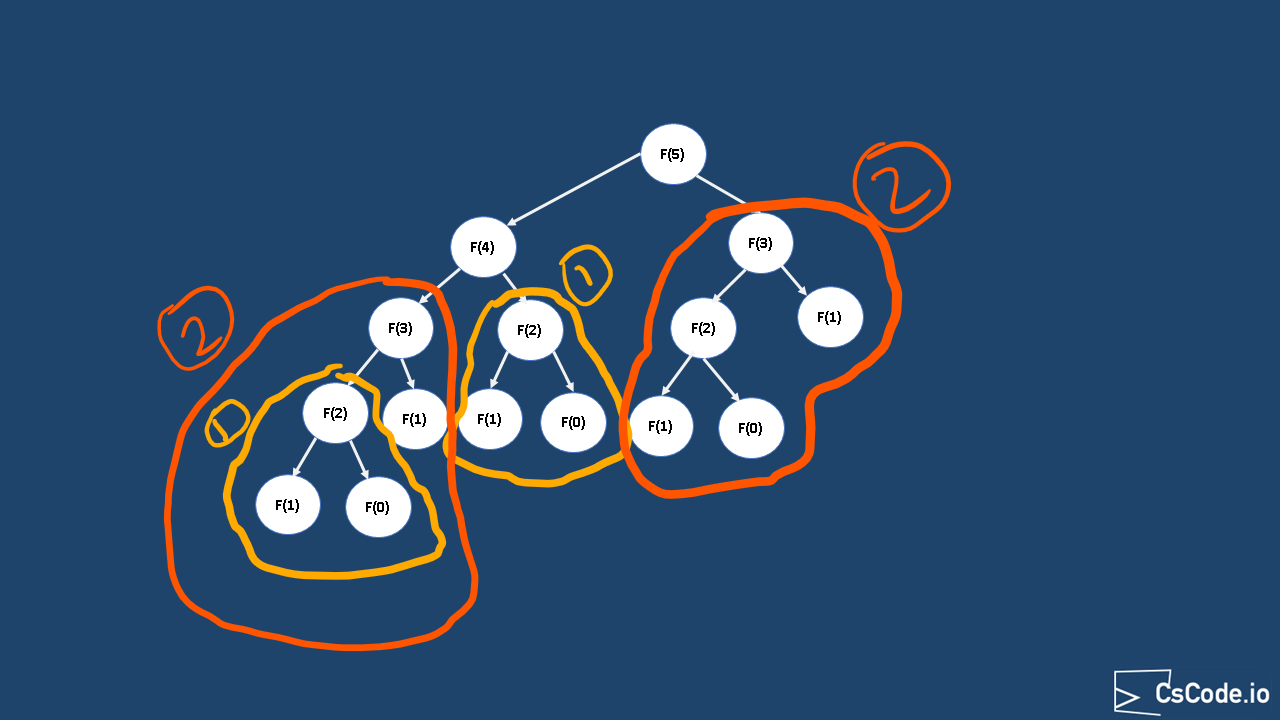
Dynamic programming can be achieved using two ways.
-
Top-Down approach:
In this approach, main problem is divided into sub problems in a recursion and cache the sub problem results
and reuse them when overlapping sub problem is repeated. This technique of caching sub problem result and re-using them
is called Memoization.
In this recursive approach we start with problem input (top) and recursively the function itself until optimal solution found to its sub problems (down). So we have to travel to the least sub problem to find optimal solution of a sub problem, which is why this approach is called Top-Down approach. This approach uses relatively more memory. -
Bottom-Up approach:
This is an iterative version of Top-Down approach, one advantage with this approach is, we start with bottom
input to start with and move towards the solution, and as we progress, we cache previously computed sub problems and re-use them.
This approach of starting with bottom input and caching results is called Tabulation technique. This approach relatives uses less memory.
We will look into solving Fibonacci problem using 4 different solutions, notice that we have come up with most optimal solution.
Let's implement solution to Fibonacci sequene using a simple recursion approach.
Complexity Analysis:
Time complexity: Above solution runs in O(2n) where
Space complexity: O(n), since we are calling the function recursively and this is the amount of memory used by stack.
In this solution, we are going to apply dynamic programing (memoization) technique, that is cache sub problem results and re-use them.
-
Create a function
fibUsingMemoization(int n) that takes input parametern and returns fibonacci of that number. -
We will create another helper function
fibRecursive(int n, HashMap<Integer, Integer> cache) , we are creating this helper function to call itself with smaller sub problems and pass the cache reference to them. -
We call this fibUsingMemoizationRecursive recursively for
n-1 andn-2 , until bases are hit or answer is found in the cache, if the answer is not found in cache then we will add it to the cache.- base cases are when input is 0 or 1, it returns 1.
Complexity Analysis:
Time complexity: After applying Dynamic programming memoization technique, we have brought down run time to O(n) where
Space complexity: O(n) as we are making recursive loops
In this solution, we are going to apply dynamic programing (tabulation) technique, that is cache sub problem results and re-use them.
-
Create a function
fibonacciUsingTabulation(int n) that takes input parametern and returns fibonacci of that number. -
Then, we will create a temporary array with size
n+1 to cache results as we compute and use them later. The cache array size is n+1 because we are going to store fibonacci of 0 as well and use them later. -
The value at ith index in the cache array represents fibonacci of number
i . So to start with we will pre-populate 0th and 1st index with 1 as these are base cases. -
Then, iteratively solve fibonacci of next number by looking at previous two values from temporary cache array,
once calculated update the cache array as well.
cache[i] = cache[i-1] + cache[i-2]; - Finally, our answer will be at nth index in the cache array, so just return that.
Complexity Analysis:
Time complexity: Tabulation approach runs in O(n) where
Space complexity: O(n), since we are storing computed values into a temporary array which is of size n.
If you notice above tabulation approach, it takes relatively less memory compared memoization technique, because this approach does not use recursion stack.
For illustration purpose, we have looked at both memoization and tabulation techniques. But, the tabulation approach can be further optimized in terms of memory. If you notice the tabulation approach, when calculating fibonacci values, we just need previous two values only, so we can cache just previous two values.
Complexity Analysis:
Time complexity: Above approach runs in O(n) where
Space complexity: O(1), since we are storing previous two values.
Above discussed examples source code can be found at