You are given an integer array
Return the fewest number of coins that you need to make up that amount. If that amount of money cannot be made
up by any combination of the coins, return
You may assume that you have an infinite number of each kind of coin.
Output: 3
Explanation: "11" can be made up of using least number of coins 5 + 5 + 1.
Output: -1
Explanation: "3" cannot be made up of using given coins.
Output: 0
Explanation: There are 0 ways to make up amount 0.
Contents
Solution 1 - using brute force approach Solution 2 - using DP memoization Solution 3 - using DP Bottom-Up approach using tabulation and memory optimization
In this approach, we will generate all possible ways to to come up with the
If the remainder becomes
For example, consider if the
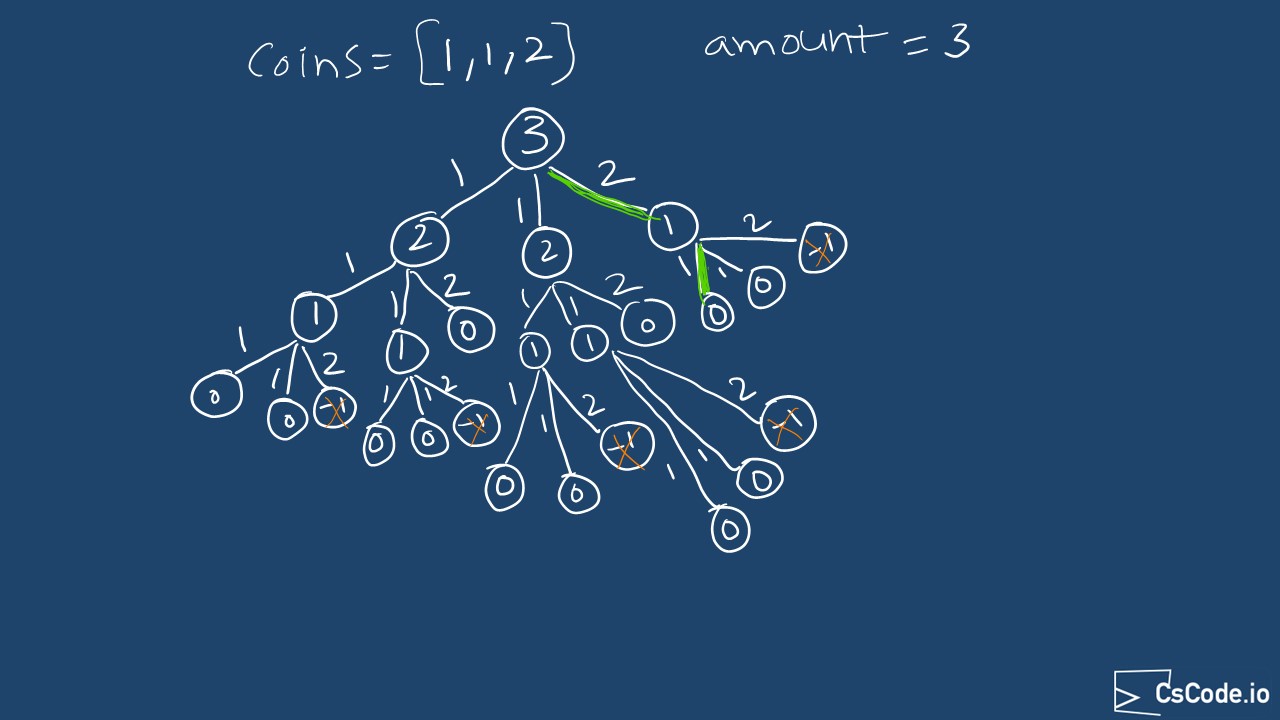
-
We will create a function
bruteForceApproach that takes inputscoins array andamount and returnsmin as answer. -
Base cases are:
-
If the input
amount is0 , then we will return0 . -
If the input
amount is less than0 , then we will return-1 as a way to indicate that we cannot make up amount using the coins given.
-
If the input
-
Then, for every number in
coins array compute the remainder usingamount - coins[i] , and callbruteForceApproach function recursively withcoins array andremainder . Repeat this process until one of the one of the base case is hit. i.e. remainder will become 0 if we can make up amount or remainder will become less than 0 if the amount cannot be made using coins. -
While calling the
bruteForceApproach function recursively, if a path found to make up the sum, that is return value of the function will be greater than or equal to 0, then increment the count of coins and keep track ofmin variable.
Complexity Analysis:
Time complexity: Above code runs in O(2t + n) time where
Space complexity: O(t + n) since we calling the function recursively, this is the stack memory.
From the above brute force approach, we can notice that there are some overlapping problems, such as sub problems highlighted in the below diagram, likewise there are many other overlapping sub problems.
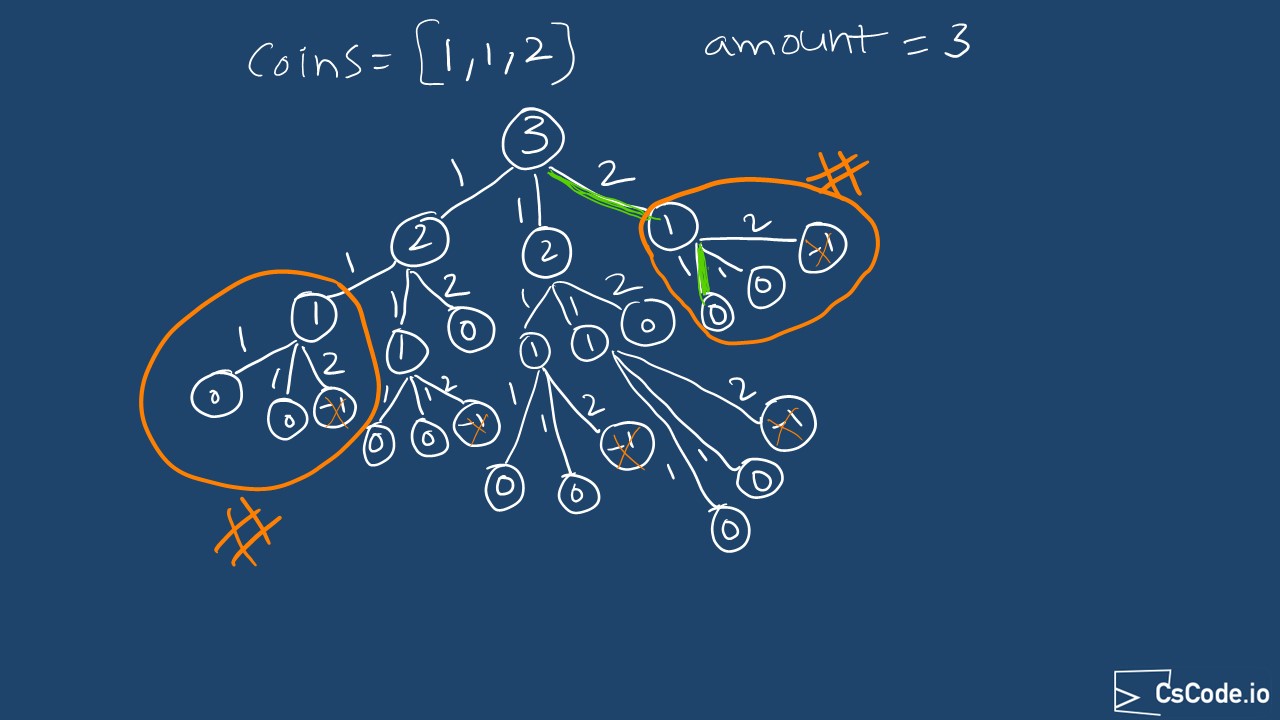
In this approach, we are going to cache the results in a
Complexity Analysis:
Time complexity: Above code runs in O(t * n) time where
Space complexity: O(t * n) for the stack used in recursive calls and the cache that we are maintaining.
In this approach we will look at solving the problem using Dynamic programming using tabulation caching mechanism.
The time and memory complexity will be same as above solution using memoization, but we can optimize memory in this approach.
In this solution, we are going to create a temporary array to cache minimum number of coins needed to make up
-
We are going to initialize
cache[0] = 0 , that is to make up amount0 we need0 coins and initialize other indexes withamount + 1 as a way to indicate whether we can make up that amount or not.int[] cache = new int[amount +1]; // +1 to include 0 amount as well. cache[0] = 0; Arrays.fill(cache, 1, amount+1, amount+1); // here, we are passing array to be filled, starting index, // end index (exclusive) and value to be filled -
When we are at index
i (here i represents an amount), we check how many coins needed to come up with that amount:-
For every coin from the
coins array, check if the remainder amounti - coin is greater than equal to 0, meaning that it is a considerable coin to make up the indexi amount. -
Update the cache with
MIN (cache[i], 1 + cache[i-coin]) , here we are checking1 + cache[i-coin] to check if the remainder amounti-coin already present in cache and if that is minimum, we take that.cache[i] = Math.min(cache[i], 1 + cache[i-coin]);
-
For every coin from the
-
Finally, if the value at
amount index in cache array is notamount +1 (what it is initialized with), then simply return that, otherwise return-1 to indicate that amount cannot be made up using coins.
Consider an example with
-
In the first step we will initialize cache array with
cache = [0, 4, 4, 4] . -
When
i == 1 , that is for amount 1:-
Check if we can consider 1st coin
1 , remainder will be 0, so we can consider it. Now update the cache.cache[1] = MIN (4, 1 + cache[remainder]) = MIN (4, 1 + cache[0]) = MIN(4, 1 + 0) = 1; -
Check if we can consider 2nd coin
1 , remainder will be 0, so we can consider it. Now update the cache.cache[1] = MIN (1, 1 + cache[remainder]) = MIN (1, 1 + cache[0]) = MIN(1, 1 + 0) = 1; -
Check if we can consider 3rd coin
2 , remainder will be -1, so we cannot consider this coin as this coin is greater than amount 1.
-
Check if we can consider 1st coin
-
When
i == 2 , that is for amount 2:-
Check if we can consider 1st coin
1 , remainder will be 1, so we can consider it. Now update the cache.cache[2] = MIN (4, 1 + cache[remainder]) = MIN (4, 1 + cache[1]) = MIN(4, 1 + 1) = 2; -
Check if we can consider 2nd coin
1 , remainder will be 1, so we can consider it. Now update the cache.cache[2] = MIN (2, 1 + cache[remainder]) = MIN (2, 1 + cache[1]) = MIN(2, 1 + 1) = 2; -
Check if we can consider 3rd coin
2 , remainder will be 0, so we can consider it. Now update the cache.cache[2] = MIN (2, 1 + cache[remainder]) = MIN (2, 1 + cache[0]) = MIN(2, 1 + 0) = 1;
-
Check if we can consider 1st coin
-
When
i == 3 , that is for amount 3:-
Check if we can consider 1st coin
1 , remainder will be 2, so we can consider it. Now update the cache.cache[2] = MIN (4, 1 + cache[remainder]) = MIN (4, 1 + cache[2]) = MIN(4, 1 + 1) = 2; -
Check if we can consider 2nd coin
1 , remainder will be 1, so we can consider it. Now update the cache.cache[2] = MIN (2, 1 + cache[remainder]) = MIN (2, 1 + cache[1]) = MIN(2, 1 + 1) = 2; -
Check if we can consider 3rd coin
2 , remainder will be 1, so we can consider it. Now update the cache.cache[2] = MIN (2, 1 + cache[remainder]) = MIN (2, 1 + cache[1]) = MIN(2, 1 + 1) = 2;
-
Check if we can consider 1st coin
-
Finally, check if
cache[amount] , this value is update that what it is initialized with, so simply rturn that. So, our answer is 2.
Complexity Analysis:
Time complexity: Above code runs in O(t * n) time where
Space complexity: O(t) where t is the input
Above implementations source code can be found at