Note that it is the kth largest element in the sorted order, not the kth distinct element.
Example 1
Output: 5
Explanation : after sorting above array, it will become [1, 2, 3, 4 , 5 , 6]. So 2nd largest element is 5.
Example 2
Output: 4
Explanation: after sorting above array, it will become [1, 2, 2, 3, 3, 4 , 5, 5, 6]. So 4th largest element is 4.
Note: You can assume
- In this solution we are going sort the array first, then simply get
array.lenth - k th element (i.e. taking Kth element from bottom after sorting in incremental order).
Complexity Analysis:
Above code runs in O(n log n) time complexity where
This solution is definitely a overkill to sort entire array for getting just one number.
- In this solution we are going use an intermediate data structure, Min Heap
(
PriorityQueue in Java) to store array elements. - Min Heap works such a way that, it always keeps the
min element at the top of Queue. So, what we are going to do is whenever Min Heap is filled with K elements, then remove the top element when a new element is inserted to ensure that Min Hep has only K elements at any given point of time and Kth largest stays at top.
-
As you loop through elements in the array, add them to PriorityQueue using
PriorityQueue.add(element) -
Then, check if the PriorityQueue already filled with K+1 elements, then remove the top element using
PriorityQueue.poll() , leaving exactly K elements in the Heap.
- Once we visited all elements in array, simply return the top element using
, which is nothing but Kth largest element.
Complexity Analysis:
Above code runs in O( n log k) time complexity where
Min Heap runs a procedure called
This is better than previous solution if K is reasonably less than N.
- The main idea behind this solution is to use
Quickselect algorithm, it uses the same technique as Quicksort algorithm and find Kth largest element. - Similar to Quicksort algorithm, we will choose an element from the array as pivot and partition the array such that all the elements smaller than pivot are aligned to left
and greater than pivot are aligned to right side.
This is achieved using
method in below code. - Once the array is split with
pivot element in the middle, resultant array looks like below diagram. It is also true that the pivot element is correctly positioned to its place if the array is fully sorted. - Now, we can draw three conclusions from above.
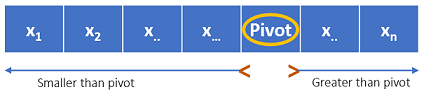
- If pivot's index is K, then we have found our answer.
-
If pivot's index is less than K, then our answers in on left side of the array.
So, repeat above steps on pivot's left side sub array
[left,.....,pivot-1] . -
If pivot's index is greater than K, then our answers in on right side of the array.
So, repeat above steps on pivot's right side sub array
[pivot+1,.....,right] .
Let's understand this with an example
Since we have to find 2nd largest element and the input array size is 7, so 2nd largest element is nothing but 5th indexed element after array is sorted.
Step 1: We are going to choose right most element as pivot, that is 6.
Step 2: Using 6 as pivot element, move all elements smaller than 6 to left side and greater than 6 to right side of array using
compare and swap technique, so the resultant array looks like this
[3,2,1,5, 6 ,9,8].
If you take a closer look at the resultant array after step 2, pivot element is positioned at index 4,
which is the correct position when the array is fully sorted.
Step 3: Now, draw one of below conclusions.
- Is the pivot's new position is K ? if YES then we have found our answer, but pivot's position is 4, but we are looking for 5th indexed element from sorted array.
-
Now, we know for sure our answer will be between index 4 and 6.
So, repeat above steps for this sub array
[4,...,6] and at one point, pivot's position will be equal to K.
After step 3, we can eliminate left partitioned array and just continue above three steps using this new sub array with indexes [pivot_index+1,.....,right].
Complexity Analysis:
QuickSelect algorithm runs in O(n) time complexity in average case where
This procedure is an in-place algorithm, so it takes O(1) constant space.
Partition algorithm illustration:
For the illustration purpose, let's take input array as [3, 2, 1, 9, 8, 5, 6] and K = 2. So Kth largest element is nothing but
Input Array :
Step 1 is to pick a pivot element, we are choosing right most element, that is pivot = array[right] = 6.
Step 2 initialize two variables
- If the element is less than pivot then swap storeIndex element with leftIndex element that is currently at, and increment storeIndex and leftIndex.
- If the element is greater than or equal to pivot then don't swap elements, increment leftIndex and continue with next element.
- In 1st iteration, check
array[leftIndex] < pivot , that is3 < 6? this is true, so swap array[leftIndex] and array[storeIndex], since left index and storeIndex are same, after swapping resultant array will be same [3, 2, 1, 9, 8, 5, 6]. Now increment storeIndex, sostoreIndex = 1 - In 2nd iteration, check
array[leftIndex] < pivot , that is2 < 6? this is true, so swap array[leftIndex] and array[storeIndex]. After swap resultant array will be same as input array and storeIndex will be incremented to 2. - In 3rd iteration also, array will be same as input array after swapping and storeIndex will be incremented to 3.
- In 4th iteration, check
array[leftIndex] < pivot , that is9 < 6? this is false, so we will not swap elements and storeIndex is not touched. - In 5th iteration, check
array[leftIndex] < pivot , that is8 < 6? this is false, so we will not swap elements and storeIndex is not touched. - In 6th iteration, check
array[leftIndex] < pivot , that is5 < 6? this is true, so swap array[leftIndex] and array[storeIndex]. After swap resultant array will look like [3, 2, 1, 5, 8, 9, 6] and storeIndex will be incremented to 4. - We have come to end of loop, now swap storeIndex and right index (pivot element) to complete the partitioning and the array now looks like this [3, 2, 1, 5, 6, 9, 8].
- Now, check where the pivot element is positioned at (storeIndex) and compare this with K, pivot is now at 4th index, but we are looking for 5th indexed element after sorting.
- At this step we know for sure our answer is on right partition of the array, so we will continue all the steps described above with
leftIndex as 5 andrightIndex as 6.
Once the array goes through
Above examples source code can be found at